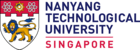
Nanyang Business School Forum on Risk Management and Insurance
Credit Rating Migration Risk and Interconnectedness in a Corporate Lending Network
Tags: credit rating migration risk, corporate lending, interconnectedness, centrality measure, credit value at risk and expected shortfall, IRFRC
More from: Masayasu Kanno
This study is about credit rating migration risk and interconnectedness in a corporate lending network. A large body of financial literature exists on corporate lending in countries worldwide. However, the literature barely mentions the interconnectedness of corporate lending contracts in a country. A network analysis of contractual relationships in the corporate lending network has yet to be conducted. It is important to analyze the credit rating migration risk in the lending network.
This study conducts three analyses: credit risk analysis, network analysis, and stress test.
In terms of credit risk analysis, this study uses firm-level outstanding lending contracts with borrowers and lenders names. The dataset covers almost all lending contracts of bank-to-listed firms and insurer-to-listed firms. Thus, it is to be noted that the dataset is quite rare and valuable in the world. In addition, this study uses two risk measures such as VaR and ES, and two tail dependencies expressed in Gaussian Copula and t-Copula.
The credit risk exposure size in the entire network remain almost unchanged from FY2008 to FY2009 despite the GFS. In addition, major banks and other large banks have a share of 50% to 59% of the total amount less unknowns. By contrast, regional banks (I and II) have an almost constant share of 9% to 12%, and insurers have a share of 8% to 14%. Consequently, by applying the same 99.9% confidence level to selected institutions, Gaussian copula VaR, Gaussian copula ES, t copula VaR, and t copula ES are ranked in ascending order for the institutions. t copula ES is 3.48 times as large as Gaussian copula VaR for the Mizuho Bank and 3.37 times larger than the average of 16 institutions. As a result, ES requires more capital than VaR for the institutions.
In addition, the distributions of most banks are nearly asymmetrically unimodal distributions, whereas the distributions of some insurers are bimodal distributions. The average coverage pertaining to outstanding lending data in the database is 27% for all city banks, 36% for major trust banks, 5% for all regional banks, and 18% for major life insurers. Because credit risk amounts for three mega banks are JPY 159—223 billion based on Gaussian copula VaR and JPY 657–828 billion based on t copula ES, the figures need to be monitored carefully.
In terms of network analysis, this study calculates network statistics and four selected centrality measures such as degree centrality, eccentricity, HITS hub centrality, and eigenvector centrality. In the top 20 ranking of degree, they include 15–17 banks and 2–4 insurers. Because no firm borrows money from hundreds of institutions, no firm ranks in the top 20.
In terms of stress test, this study examines the increase of credit risk by lending assets deterioration in the future. Empirical default probabilities of Japanese firms rated as speculative grades reached a peak of 15% during two years following LB’ bankruptcy. Hence, this test considers that the historical economic scenario pertaining to the credit rating migration matrix just after LB’ bankruptcy is one of the worst scenario cases faced by Japanese economy. The transition matrix for FY2008–FY2009 stands out w.r.t. large downgrading probabilities, such as 43% for a downgrade of a BB rating to default and 50% for a downgrade of a B rating to default. Other parameters such as factor correlation matrix are assumed to be the same as those at the end of March 2016.
Consequently, because many defaults occur, the lending network becomes much sparser. Defaulted firms account for 1.7% of all Japanese listed firms in terms of Gaussian copula dependency and 2.2% in terms of t5 copula dependency. Stressed VaR and ES are over around 500 times greater than normal. In particular, t5 copula ES of JPY 38 trillion is an enormous sum in Japanese financial system.
At the same time, from two directed graphs of Gaussian copula dependency and t5 copula dependency of all non-defaulted firms, each graph shows a sparser network structure. In particular, because t5 copula brings tail dependency into the value distribution, t5 VaR and t5 ES are much larger than Gaussian copula VaR and Gaussian ES respectively.
First, this study evaluated credit risk exposure for all Japanese listed firms. The values of outstanding lending of mega banks also substantially reduced just after LB bankruptcy. By contrast, outstanding values for life insurers increased after FY2009. The analytical results show that banks are affected by capital requirement of Basel II and III, whereas life insurers aimed to improve their investment performance during the studied period.
Second, this study measured the portfolio credit risk of corporate lending exposure for major banks and other large banks, and major life insurers. In particular, ES is expected to ensure the prudent capture of tail risk and has actually been introduced in insurer solvency regulation, as illustrated by the SST. In addition, the choice of copula is critical for correctly measuring the dependence between systematic risk factors.
Third, this study analyzed the network structure of corporate lending among bank-to-listed firms and insurer-to-listed firms using major centrality measures. Banks and insurers play a central role in terms of degree centrality. However, degree centrality decreased gradually after the GFS. This may mean a decrease in the number of CPs.
Fourth, this study conducted a stress test in terms of network structure. Because 1.7% of all Japanese listed firms defaulted in terms of Gaussian copula dependence and 2.2% in terms of t5 copula dependence, the network structure became much sparser.
Finally, this study’s analyses on CRM risk and interconnectedness in a lending network can serve as warnings to related entities such as supervisory authorities and financial institutions about risk perception.
The complete paper is available for download at:
https://ssrn.com/abstract=3152762.
0 Comments