test
Archives
Chapter 20
Testing stone tools
Stone tools and language evolution
Chapter 20 – Stone Tool Technology and Language Evolution
2019: Anthony Tan, Kerlyn Fu, Ryan Lim
1. Introduction – Stone Tools in Hominin Evolution
The first evidence for tool production dates from about 2.5 million years ago (mya), though controversial examples dating back to even further back are aplenty. The onset of obligatory stone tool use after 0.3 mya may be linked to the evolution of spoken language (Shea, 2017). Stone tool-making is a deliberate act; each resulting tool is thus a snapshot of hominin behavior and an important material phenomenon we can use to contextualize claims of cognitive change against. Adopting stone tool technology thus made a difference to our survival prospects as large populations were not only capable of sustaining innovations, but using them to expand and adapt to new environments (Davidson, 2016).

1.1 Development of stone tools
Complex tool use and language are unique characteristics of the human species and contains widespread evidence of human behavior (Shea, 2017). Archaeological records demonstrate that the first recognisable tools were made from organic material decompose e.g. stone. A scheme of tool classification was devised by Grahame Clark (1969), that distinguishes the five different modes. Modes cannot be chronologically divided per se, rather, they reflect the groupings of tools that share distinctive morphological characteristics (Foley & Lahr, 2003). As such, the implementation of one mode at a certain point in time is not a universal affair – an older mode might have been replaced in one region, but continue to be used in another.

As the last two modes are only well attested in the final stages of the Upper Paleolithic (50kya onwards), the earliest three modes are the most pertinent for our focus on language emergence.
1.2 Oldowan tools (Mode 1)
Mode 1 represents the simplest mode of production, which is Oldowan stone tools. Tools are made by using a hammerstone to strike flakes off a core – if successfully flaked, the sharp edge of a flake becomes a cutting tool. The number of flakes could vary, but what held this system of production together was the simple platforms and lack of preparation involved. This mode resulted in relatively little diversity of toolform and ease of preparation of the striking platforms.

Oldowan tool-making demands little in terms of cognition as stone tool producers are only required to visually identify ideal platforms and remove flakes until the core is exhausted. Oldowan tool manufacture requires the coordination of visual attention and motor control to successfully remove simple flakes. Experimental findings reveal that ape-like cognition was the minimum to produce Oldowan tools 1.8mya, whereas human-like cognition in modern knappers is not fully recruited in knapping (Shea, 2013).
1.3 Acheulean tools (Mode 2)
Acheulean tool production spanned over 1.5my and produced some of the most significant artefacts, including the characteristic handaxe. (Beyene et al., 2013). The Acheulean handaxes were produced by the bifacial reduction of a block or large flake blank around a single, long axis. Acheulean handaxes are thought to have been produced by two extinct hominin species, Homo erectus and Homo heidelbergensis (Corbey, 2016).

Cognitively, the brain areas activated in Acheulean handaxe production overlaps those used in speech and language (Putt, 2017). Previous studies on Acheulean stone tool use by Putt (2017) suggest that the neural architecture for spoken language might have been in place around 1.7 Ma.
Acheulean tool-making is a process that incorporates many intermediate goals (Section 3.3) requiring the cooperation of higher-order motor planning, working memory, and auditory feedback mechanisms to attend to information from multiple modalities (Putt et al., 2017). In order to produce the thin bifaces, Archulean knapping employs techniques such as platform faceting and raising the plane of the intersection, taking small strikes at a steep angle on the surface, followed by a large strike at a shallow angle on the opposite surface (Shipton, 2018). Information from multiple modes must be considered as well. For example, knappers must distinguish between different sounds produced during knapping and relate such sounds to the different stages of making a handaxe.
Interestingly, the Acheulian technology coincides with the evolution of a derived middle ear anatomy in Homo that was more attuned to human speech frequencies (Quam et al., 2013). Acheulian knapping may have contributed to the evolution of this auditory function, and perhaps facilitated the evolution of neural connections involved in speech perception. Putt et al., (2017) also suggested that the working memory might have played a role in early Acheulean tool-making, which is also important for the learning of other complex behaviours.
1.4 Mousterian tools (Mode 3)
The Mode 3 stone technology represents a major shift in the production of lithic stone tools, although it shares with some elements of tool production with Mode 2. The earliest Mode 3 tools began appearing 500kya. The key difference is that in the production of Mode 3 tools, the core is prepared prior to the striking off of a major flake so as to have greater control over the flake’s thickness and shape. The Levallois technique (Figure 5) is one well-attested technique, where a core is treated and prepared in a much more sophisticated manner than its Acheulean predecessor. Although the cognitive basis for Mode 3 tool-making shares much in common with the production of Mode 2 handaxes, products of Mode 3 tool-making are much more diverse and specialized in function, with a greater emphasis on smaller items.
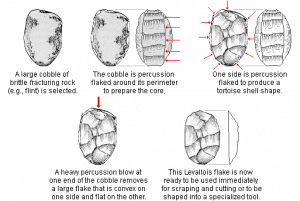
2. The Technological Origins of Language
The technological hypothesis (TH) does not refer to a single specific or empirically testable regarding the evolution of language, rather, it refers to a loose collection of explanations which identify technology development as the critical spark for the linguistic faculties of our hominin ancestors.
Since language emerged parallel to, if not as a consequence of, tool development, TH rejects propositions that our language faculty began as an inherently complex phenomenon. For example, TH is incompatible with the single random mutation put forth by Chomsky & Berwick (2016). One direct consequence is that complex linguistic structures can only be posited to have emerged after Oldowan technology. Oldowan technology was characterized by an extended period of stagnation, which persisted over 700ky and until the emergence of Acheulean tools 1.7mya (Lepre et al. 2011); hence no technological trigger for complex forms of communication should be found in the Oldowan era.

2.1 Exaptation
In evolutionary theory, an exaptation is a trait which originally evolved to serve one particular function, but has since then evolved to serve another distinctly unrelated function.
A frequently cited example of an exaptation is the evolution of bird feathers: the discovery of bird feather structures in dinosaurs, which were incapable of flight, prompted biologists to propose that bird feathers were originally designed for attracting mates or for temperature regulation. Somewhere along the way and after the extinction of dinosaurs, they were co-opted by current species for flight.

TH similarly incorporates notions of exaptation by proposing that technologically skilled hominins were selected for certain capabilities, which were then repurposed for communicative, and ultimately linguistic functions. In this sense, language co-opted or even ‘hijacked’ the initial tool-making purposes of these technological capabilities.
2.2 Gesture versus speech
While most TH proponents identify our language capabilities as an exaptation of stone tool capabilities, there is less consensus on the kind of language that developed. Corballis (1992a, 1992b) suggests that one possibility for the nature of “proto-language” is that it was fundamentally gestural in nature. According to Bickerton (1995), the compositional view of protolanguage hypothesizes that Homo erectus communicated by a protolanguage in which a few words were strung together without syntactic structure. It has long been argued that the origins of language lie in gesture, and it is clear from modern sign languages that speech is merely one particular manifestation of the linguistic capacity (Donald, 1991; Goldwin-Meadow, 1993; Kendon, 1993). Hence, proto-language may have involved all the elements of spoken language but was gestured rather than spoken.
Corballis (1992b) describes a possible gesture-to-speech scenario, arguing that such a transition would have freed the hands. Therefore, this laid the foundations for the eventual technical and artistic developments during the Upper Palaeolithic. While this idea appears to be unfairly dismissive of the technological achievements of the Middle Palaeolithic, Lieberman (1991, 1992) has argued that Neanderthals could only produce a very restrictive range of vocal sounds due to the relative positions of the larynx and windpipe.

Others suggest that gesture may not have emerged before speech, but perhaps in parallel (Steklis & Raleigh, 1979b). Shared neural structures in the left hemisphere (Section 3) provide strong evidence for tool use preceding and giving rise to human speech. However, speech may have evolved not out of an intermediate gestural phase, but in response to rapidly changing environmental pressures. These environmental pressures, in turn, would have led to increased development of these neural structures, laying the foundations for the control needed to articulate speech.
One means of moving the gesture versus speech debate forward is the use of experimental approaches (Section 4). These are particularly useful to infer the exact means our Paleolithic ancestors needed to transmit stone tool technology.
3. Evidence from neuroanatomy
3.1 Homology vs. Analogy
Since the study of tool-making fundamentally treats non-linguistic phenomena as primary evidence, some criteria are necessary to determine the validity of links drawn between these non-linguistic phenomena and the specific properties of language.
A key notion in evolutionary research concerns the distinction between homology and analogy. Consider the case where a property observed in tool-making seems to parallel a specific feature of human language. The challenge is then deciding if these two similar traits can be attributed to a common ancestral or phylogenetic origin (homology), or alternatively, that they lack a common origin, but parallel features have emerged as a result of similar responses to another shared circumstance (analogy).
Evidence demonstrating homologous rather than analogous links between technology and language is of much greater utility because it strengthens the plausibility of TH’s scenario where language and technology co-evolved. Even if particular traits within tool-making and language reveal a similar organization, this only amounts to an analogy. Greenfield (1991) was one of the first to introduce criteria for determining homology, suggesting that a language-technology homology requires: (i) a common neuroanatomical base, and (ii) that this base is specific to language. Subsequently, the homology-analogy distinction is a useful framework to assess the robustness of the various technology-language relationships detailed below:
3.2 Manual Praxis
In 1876, Friedrich Engels pointed out that bipedalism and language were two out of three features most important in human evolution. Much like language, learning to use our hands for fine manipulation is critical in accounting for hominin’s exceptional evolutionary trajectory, since bipedalism would have been nowhere as advantageous otherwise. As anybody who has ever attempted to pick up a pencil with their toes (rather than fingers) will attest to, it is a much easier affair controlling our hands over anywhere else. In fact, one finger roughly corresponds to an entire foot in terms of the motor precision we possess. This specialization of the hands is known as manual praxis, and it has been identified as the ability we rely on when using a pair of scissors, threading needles (Guiard 1987) or completing other non-object manipulation tasks as in all communicative gesture.
Despite the stone tools’ simple appearances, successful stone tool manufacture is a sophisticated affair for which manual praxis is critical. Knapping even the oldest Oldowan tools always involves a transformation from sensory information about the object into how the hand can best grasp the object (Frey et al. 2005) – this is a highly coordinated effort which mobilizes both hands (Ruck 2014):
- The non-dominant hand is used to manipulate the core by looking for a suitable edge angle and thus prepare it for the knapping action.
- The dominant hand makes a blow that is aimed accurately and at a minimum speed, while the non-dominant hand maintains its precise grip on the core.
Within the brain, the inferior frontal gyrus (IFG) is one productive area of study, revealing many documented overlaps between language and tool use. The linguistic significance of the IFG stems from a much older tradition beginning with Broca’s seminal identification of Broca’s area (Figure 9). The study of aphasic patients, who suffer lesions in Broca’s area, has long associated damage in Broca’s area with agrammatic speech. However, recent evidence has also been steadily connecting this with non-linguistic effects, including the inability to perform simple actions involving tool manipulation as well as inability to pantomime tool use (Kroliczak & Frey 2009). The IFG is thus being re-conceived as a ‘supramodal processor’ (Stout & Chaminade 2012) involved in translating abstract sequences into specific motor actions, be it the articulation of speech or the control of a stone core.
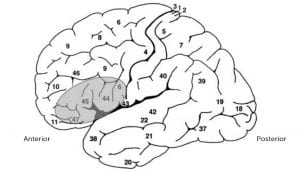
The IFG differs from Broca’s area as it extends to regions BA 47 (pars orbitalis) and BA 6 (ventral premotor cortex) of the frontal lobe. These regions are significant because it has been used to characterize gradient functioning within the IFG. The division of work across posterior and anterior IFG reflects increased linguistic complexity (Putt 2016) from phonological to syntactic and semantic domains respectively. A similar gradient has been identified in manual actions, with increased activation of BA 45 and BA 47 (most anterior) during more motorically complex manual actions (Molnar-Szakacs et al. 2006).
The IFG’s connection to tool-making becomes apparent here, because as demonstrated earlier, the evolution of tool-making also reflects increasingly complex degrees of manual praxis. What distinguishes the very first deliberate Oldowan tool manufacture from simple percussion on rocks (striking) then becomes this feature of manual praxis. Stout & Chaminade (2012) reported, in a positron emission tomography study conducted on Oldowan tool knappers, that Oldowan tool manufacture heavily demanded grasp coordination. In turn, areas such as the ventral premotor cortex (BA 6) were activated; this area had also been previously identified by Hagoort (2005) as a plausible member of the left frontal language network. Crucially, the anterior IFG lying on the opposite, abstract end of the gradient, was not recruited. Oldowan tool-making is manipulatively complex and its execution involves finely calibrated and executed motor actions. However, its structural organization is nowhere as complex that the anterior, higher-order functions are mobilized.
Linguistic Implications
Such studies have been productive in isolating the manual praxis of tool manufacture within specific neural substrates. However, conflicting explanations exist as to the linguistic counterpart, broadly diverging along the vocal versus gesture-centric interpretations of TH described earlier. For example, Stout & Chaminade (2012) suggest that above study’s results favour a vocal scenario where ‘the elaboration of a praxic system… supported the enhanced articulatory control required for speech’, might indicate that it is the precursor to the fine distinctions in human language’s feature-based phonological systems.
However, the same neural evidence of manual praxis in tool-making may also support a gestural origin of language. Fine motor control developed for tool manufacture may have been repurposed for intentional gestural communication, perhaps beginning with transmission through imitation.
Both interpretations converge in establishing tool-making’s motor demands as the prerequisites for intentional communication, be it vocal or gestural. The neural foundations Oldowan tool-making laid are fundamentally compatible with vocal or gestural outcomes; the question becomes whether voice or gesture came first (or perhaps simultaneously) (Vaesen 2012). One way to further advance this debate involves new experimental setups, which study transmission specifically aim to investigate if one mode may be more critical for passing on Oldowan technology over the other (see Section 4).
3.3 Hierarchical Organization
One of the first attempts to explicitly connect linguistic hierarchies with the general hierarchies behind object manipulation and combination was made by Greenfield (1991). Observing infants’ assembly of Russian dolls, she identified subassembly as a complex manipulative strategy where two objects are first combined before the combined object is subsequently placed into a larger one.
Subassembly, accessible by only older children, then demonstrates increasing hierarchical complexity in ontogenetic development, which is in turn reflective of manual intelligence. Likewise, the first phrases formed by children (want [more cracker]) demonstrate elementary nesting and a greater complexity over the telegraphic speech (more cracker) observed earlier. Citing Grossman (1980)’s study of aphasics and their ability to replicate hierarchically organized tree structures (Figure 10) Greenfield further located a common neural basis, first asserting that these two uses of hierarchical processing do not modularly originate from a region like Broca’s area per se. Rather, they reflect the ontogenetic development of neural circuits specialized for manual sequencing and grammar respectively.

While researchers today are more wary of accepting infant speech as a suitable parallel to the first languages (Bickerton 2003), both the hierarchical ordering of language and the goal-driven, subordinated nature of object manipulation remain widely accepted. Moreover, hierarchical ordering is not only a matter of syntax; phonological rules for combining sounds also constitute linguistic hierarchies, for example syllable structure subordinates combinations of vowels and consonants (Lieberman 1984). Such assumptions are the bases which research into tool-making, cognition and language have used to present scenarios for TH.
Research into the neurological nature of late Acheulean tool-making is particularly promising – the emergence of Acheulean tools mark the next major cognitive shift related to lithic technology, some 700ky after the beginnings of Oldowan tool manufacture (Rusk 2014). Late Acheulean technology can be distinguished from its Oldowan predecessors in the following aspects (Stout et al. 2008):
- Increased motor skills and awareness of stone morphology
- Elaborate planning and consideration of long-term objectives
- Greater diversity of implements designed to achieve specific knapping steps
For example, creating the thin, symmetrical bifacial handaxes begins with the removal of thin-flakes (bifacial edging). Before these, Late Acheulean toolmakers would prepare the striking surface through small-scale chippings (elementary percussion). What is important here is to coordinate multiple lower-layer actions, in advance preparation for the specific goal of bifacial edging. To proceed with bifacial edging right after a single preparation of the striking surface, as in linear sequences, runs the risk of making a tool with an inconsistent (and sometimes unusable) thickness. Longer-range dependencies between isolated actions thus reveal a nested organization, from which we can posit neural structures facilitating this.

In a study by Stout et al. (2011), late Acheulean tool-making produced a different set of neural activations from its Oldowan counterpart. Additional areas of the prefrontal cortex, including the pars triangularis (BA 45), participated in late Acheulean tool-making but not in Oldowan knapping conditions. Generally, increased prefrontal activation was attributed to the specific division of Acheulean tool-making labour in a layered manner. These therefore suggest the separation of tool-making sequences into preparatory actions, intentions and outcomes might demand more neural processing, which in turn overlap with layered language processing.
3.4 Lateralization and the right hemisphere
While classical thinking has long associated language and tool use with the left hemisphere, recent research is paying more attention to the right hemisphere’s contributions. Discourse-level processing, prosody and connotative meaning (Bookheimer 2002) are such instances of language-related tasks dominated by right hemisphere processes. Alongside this, new research is now uncovering the role of the right hemisphere in sequentially developing and coordinating of action sequences which are important for object manipulation.
Lateralization of function is a term used to describe work (linguistic and non-linguistic) being distributed across both hemispheres. One intriguing application of lateralization is the bimanual (i.e. use of both hands) nature of stone knapping discussed earlier. The non-dominant hand (typically the left) tends to not receive scholarly focus; nevertheless its role carries direct implications for the right hemisphere (which delivers input for the left hand). Particularly, significant correlations between non-dominant hand participation and the level of knapping expertise have been reported (Stout et al. 2008). While novice knappers are more preoccupied with the rapid striking actions on the right hand, expert knappers with substantial practice factor in precise and forceful left-hand grips and thus are able to capitalize on the RH-left hand system.
In Late Acheulean tool-making, achieving goal-directed nature of intermediate steps (Section 3.3) also relies on functions found in the right hemisphere. To achieve the thin handaxe shape, knappers must reject immediately desirable options such as continuing to thin one handaxe edge (at the expense of the other edge). Neurally, an inhibiting mechanism would motivate these, something which has been identified in the right ventral premotor frontal cortex (Aron et al. 2004). The highly segmented process of Late Acheulean handaxe manufacture thus mobilizes both left and right hemispheres.
Implications for Research
As a whole, discovering lateralized functions provides two possibilities to further investigate TH’s language-technology co-evolution scenario. The first concerns the emergence of functional descriptions of the human language faculty, in general linguistic theory.
Functionalist linguistics originates from the work of Halliday’s Systemic Functional Linguistics, and broadly assert that language is a means of interpersonal interaction rather than mere representation (Painter 2004). Accordingly, neurological emphasis should be placed beyond the grammar-constructing facilities typical of the left hemisphere, and on the interactional, social and affective dimensions of language usually processed by the right hemisphere (Rose 2006). These experimental archaeology results, by suggesting tool-making’s dependence on lateralized functions, thus provide a homologue to the same structures modern language uses to achieve its functional aims. Thus, it contributes to the possibly technological origins behind language evolution.
Additionally, unearthing a relationship between lateralization and level of expertise makes ethnographic approaches particularly attractive, as such approaches examine the specific process of teaching and transmission. Teaching and transmission are significant because they are a plausible means with which hominids took full advantage of and elaborated upon tool-making’s neural correlates. In other words, teaching is the potentially critical scaffold supporting the exaptation of technology’s neural functions by language. Section 5.3 introduces one such case study by Stout (2002), which makes use of ethnography to infer possible social behaviors governing stone tool production.
4 Experimental Approaches
According to TH, cultural transmission of tool making skills might have been involved in the manufacture of simple Oldowan tools but there was an unclear relation between speech evolution and stone tool making (Cataldo et al., 2018). Based on the premise that tool making is a hands-on activity, instruction by gesture would seem to be a preferred mode of teaching aid over verbal instructions.
4.1 Experimental Design
The study by Cataldo (2018) premised on the hypothesis whether verbal instruction is most effective in skills transmission. The experiment investigates the effectiveness of speech as a teaching aid during experimental transmission of the Oldowan-style tool making by comparing the flintknapping performance of subjects. The transmission efficiency of tool-making skills was compared under the four conditions: gesture group, speech group, full language group as well as a no instruction group. The full language group (gesture plus speech) would represent the current mode of human communication and a no-instruction group was included to serve as a control. Flake measurements were recorded and statistical analyses were performed.
4.2 Results and Discussion
The experiment revealed significant differences across the flintknapping performance in the four communication groups. The speech group (purple) performed significantly worse than the full language group and no difference from the control group that received no instruction. Despite the richness of the linguistic resource, speech appeared to be a poorer instruction aid in comparison to gesture. The results did not support the hypothesis, and in fact, suggest the otherwise. Speech was not an effective form of cultural transmission and lithic production might not be the triggering factor that caused speech evolution.

The experiment revealed that speech was not the most effective way in cultural transmission of Oldowan-style tool-making skills as no distinction was found between the performance of subjects in the non-instruction group and the group that received verbal instructions. This might suggest that the evolution of gesture preceded speech as speech could not have evolved in earlier tool-making process given its ineffectiveness.

Questionnaires revealed that the participants in the speech group (purple) ranked the lowest amongst the other instructional groups – gesture (orange) and full language (red). Speech was rated as a less satisfactory than gesture as a teaching aid, indicating that speech is not the most desirable form of instruction due to its inefficiency in the transmission of skills.
Therefore, judging the satisfaction of the full language group, the addition of verbal instruction with gestures did not increase the flintknapping performance or satisfaction (in comparison to the gesture group). Hence, an alternate view can be formed against TH. The experiment revealed that gestures could have possibly preceded speech to facilitate tool making in early hominins, while speech may have emerged in parallel with gesture at a later stage. This might be the explanation to the possible distinct communicative functions and how the full language evolved to be the default mode of communication in Homo sapiens.
5 Shared social contexts
5.1 The relationship between language and shared social contexts
Under the view that language and stone tool making are related by their shared social contexts, such a relationship implies that language and tool-use capabilities provided a complementary context for coevolution (Stout, 2010). Scientists, such as Kevin Laland (2017), who take on such a view argue that linguistic behaviour and Palaeolithic technologies (such as stone tools) should depend on similar cultural learning mechanisms for their reproduction. For Laland (2017), such a mechanism primarily involves teaching.
5.2 Teaching as a mode of cultural transmission
To evaluate between competing theories of the original function of language, work by Szamado & Szathmary (2006) and Bickerton (2009) have established six criteria to judge these theories and their merits relative to each other. Laland (2017) adds one further criterion of cultural niche construction, to produce seven benchmarks to evaluate alternative explanations for the original adaptive advantage of early language.
These seven constraints are (Laland 2017):
- The theory must account for the honesty of early language.
- The theory should account for the cooperativeness of early language.
- The theory should explain how early languages could have been adaptive from the outset.
- The theory should explain symbol grounding (i.e., the means by which early words acquired their meanings).
- The theory should explain the generality of language
- The theory should account for the uniqueness of human language
- The theory should explain why communication needed to be learned
How teaching satisfies the seven constraints
According to Laland (2017), teaching satisfies these seven criteria. First, if language evolved to teach relatives, then we would expect it to accurately represent information such that it is honest, since inaccurate instruction would be a waste of the tutor’s time and effort. For example, if words are easy and cost-free to produce (i.e. talk is “cheap”), then anyone is unlikely to believe what others say and there would be no incentive to learn from others if one cannot be confident that the words of others convey an accurate message. Second, if language evolved to teach solutions to problems faced by our ancestors, then language had emerged in contexts that required cooperation. Third, if cooperative traits are adaptive from the outset, then so is teaching, provided that the traits being taught are adaptive and require cooperation to function. Subsequently, teaching could be extended to support other cooperative (and adaptive) processes, such as trade and hunting. Fourth, language’s beginnings in a teaching context requires the ability to make and convey meaning. Simple attention-grabbing commands, such as pointing and gesturing, have been proven to help facilitate social learning (Tomasello, 1999). Fifth, teaching can explain language’s power to generalize information since teaching can be applied to many skills that are difficult to learn, such as foraging procedures, food-processing methods, and hunting skills (Stringer and Andrews, 2005). Sixth, the uniqueness criterion is also met because no nonhuman animals evolved language to the point that it can be used in extensive teaching—only in humans did language, teaching and cumulative culture coevolve. Last but not least, not only do cultural practices—like tool-making—needed to be learned by the next generation, it is also subject to variation. In the case of stone tools, we are talking about guided variation which entails improvements to the stone tools (e.g. reducing costs and increasing its benefits).
5.3 Case study: The Last Stone Axe Makers
Nearly 10,000 years ago, all human societies had made and used stone tools in one form or another, but in modern times the advance of more complex technologies has left few remnants of such stone tools. However, anthropological work by Toth et al (1992) and Stout (2002) on contemporary Stone Age craftsmen—particularly on the stone axe makers of the village of Langda in Indonesian Irian Jaya—suggest that stone axe production is a social phenomenon, defined by mentor-apprenticeship relations, social norms, and mythic significances.

Unlike Mode 1 and 2 stone tools, these stone axes produced by the people of Irian Jaya are more complex, and can be considered, under Clark’s (1969) to be Mode 5 stone tools, which are marked by the integration of other materials (such as wood or bone) into the stone tool. Further, the stone axe makers of Irian Jaya requires communication which is involved not only in naming the stone axe, but in teaching and instruction. For instance, Stout (2002) noted that the Irian Jaya craftsmen have subdivided the stone axe heads into many distinct parts (Figure 14). Each stone axe head also receives an ancestor name and a name to signify its “place of origin” (Stout 2002). This means that stone axe making in Langda embodies a large number of terminology and other technical concepts to learn, and so the stone axe production in Langda requires that experienced stone axe specialists pass on their knowledge to their apprentices. Hence, the manufacture of stone axes is considered a social phenomenon where the social structure, rules, and norms provide a framework for the process of learning the art of stone tool making (Toth et al 1992; Stout 2002).
6. References
Aron, A., Robbins, T.W., & Poldrack, R.A. (2004). Inhibition and the right inferior frontal cortex: One decade on. Trends in Cognitive Sciences, 8(4), 170-177.
Berwick, R. C., & Chomsky, N. (2016). Why only us: Language and evolution. MIT press.
Bickerton, A. (2009). Adam’s Tongue. New York, NY: Hill & Wang.
Bookheimer, S. (2002). Functional MRI of Language: New Approaches to Understanding the Cortical Organization of Semantic Processing. Annual Review of Neuroscience, 25, 151-188.
Bickerton, D. (2003). Symbol and Structure: A Comprehensive Framework for Language Evolution. Morten H. Christiansen and Simon Kirby (Ed.), Language Evolution (pp. 416). Oxford University Press.
Cataldo DM, Migliano AB, & Vinicius L (2018) Speech, stone tool-making and the evolution of language. PLoS ONE 13(1): e0191071. https://doi.org/10.1371/journal.pone.0191071
Clark, G. (1969). World Prehistory: A New Outline. Cambridge University Press.
Corbey, R., Jagich, A., Vaesen, K., & Collard, M. (2016). The acheulean handaxe. Evolutionary Anthropology: Issues, News, And Reviews, 25(1), 6-19. doi: 10.1002/evan.21467
Davidson I. (2016). Stone Tools: Evidence of Something in Between Culture and Cumulative Culture. In: Haidle M., Conard N., Bolus M. (eds) The Nature of Culture. Vertebrate Paleobiology and Paleoanthropology. Springer, Dordrecht.
Foley, R., & Lahr, M. (2003). On stony ground: Lithic technology, human evolution, and the emergence of culture. Evolutionary Anthropology: Issues, News, And Reviews, 12(3), 109-122.
Frey, S.H., Vinton, D., Roger, N. & Grafton S.T. (2005). Cortical topography of human anterior intraparietal cortex active during visually guided grasping. Cognitive Brain Research, 23(2-3), 397-405.
Greenfield, P.M. (1991). Language, tools and brain: The ontogeny and phylogeny of hierarchically organized sequential behaviour. Behavioral and Brain Sciences, 14, 531-595.
Grossman M. (1980). A central processor for hierarchically-structured material: evidence from Broca’s aphasia. Neuropsychologia, 18(3), 299-308.
Guiard Y. (1988). Asymmetric division of labor in human skilled bimanual action: the kinetic chain as a model. J Mot Behav, 20(3), 374.
Hagoort P. (2005). On Broca, brain, and binding: a new framework. Trends Cogn Sci, 9(9), 416-423.
Króliczak, G., & Frey, S.H. (2009). A common network in the left cerebral hemisphere represents planning of tool use pantomimes and familiar intransitive gestures at the hand-independent level. Cereb Cortex, 19(10), 2396-410.
Laland, K.N. (2017). The origins of language in teaching. Psychonomic Bulletin & Review, 24(1), 225-231.
Lepre C.J. et al (2011). An earlier origin for the Acheulian. Nature, 477, 82-85.
Lieberman, P. (1987). The Biology and Evolution of Language. Harvard University Press.
Molnar-Szakacs, I., Kaplan, J., Greenfield, P.M., & Iacoboni, M. (2006). Observing complex action sequences: The role of the fronto-parietal mirror neuron system. NeuroImage, 33(3), 923-935.
Painter, C. (2004). Learning How to Mean: Parent-Child Interaction. Tom Bartlett and Gerard O’Grady (Ed.), The Routledge Handbook of Systemic Functional Linguistics (pp. 682). Routledge.
Putt, S. (2016). Human brain activity during stone tool production: tracing the evolution of cognition and language (Doctoral dissertation). Retrieved from University Archives at the University of Iowa.
Putt, S., Wijeakumar, S., Franciscus, R., & Spencer, J. (2017). The functional brain networks that underlie Early Stone Age tool manufacture. Nature Human Behaviour, 1(6). doi: 10.1038/s41562-017-0102
Quam, R., de Ruiter, D., Masali, M., Arsuaga, J., Martinez, I., & Moggi-Cecchi, J. (2013). Early hominin auditory ossicles from South Africa. Proceedings Of The National Academy Of Sciences, 110(22), 8847-8851. doi: 10.1073/pnas.1303375110
Rose, D. (2006). A Systemic Functional Approach to Language Evolution. Cambridge Archeological Journal, 16(1), 73-96.
Ruck, L. (2014). Manual praxis in stone tool manufacture: implications for language evolution. Brain Lang, 139, 68-83.
Shea, J. (2017). Occasional, obligatory, and habitual stone tool use in hominin evolution. Evolutionary Anthropology: Issues, News, And Reviews, 26(5), 200-217. doi: 10.1002/evan.21547
Shipton C. & Nielsen M. (2018) The Acquisition of Biface Knapping Skill in the Acheulean. In: Di Paolo L., Di Vincenzo F., De Petrillo F. (eds) Evolution of Primate Social Cognition. Interdisciplinary Evolution Research. Springer.
Stout, D. (2002). Skill and Cognition in Stone Tool Production: An Ethnographic Case Study from Irian Jaya. Current Anthropology, 43(5), 693-722.
Stout, D., Toth, N., & Chaminade, T. (2008) Neural correlates of Early Stone Age tool-making: technology, language and cognition in human evolution.. Philos Trans R Soc Lond B Biol Sci, 363(1499),1939-49.
Stout, D. (2010). Possible Relations between Language and Technology in Human Evolution. April Nowell, Iain Davidson (Ed.), Stone Tools and the Evolution of Human Cognition (pp. 159-184). Boudler: University Press of Colorado.
Stout, D. and Chaminade, T. (2012) Stone tools, language and the brain in human evolution. Philos Trans R Soc Lond B Biol Sci, 367(1585), 75-87.
Stringer, C., & Andrews, P. (2005). The complete world of human evolution. London: Thames and Hudson.
Szamado, S., & Szathmary, E. (2006). Selective scenarios for the emergence of natural language. Trends in Ecology and Evolution, 21, 555–561.
Thompson, H. (2019). The Oldest Stone Tools Yet Discovered Are Unearthed in Kenya.
Retrieved from https://www.smithsonianmag.com/science-nature/oldest-known-stone-tools-unearthed-kenya-180955341/
Toth, N., Clark, D. & Ligaue G. The Last Stone Ax Makers. Scientific American, 267(1), 88-93.
Tomasello, M. (1999). The cultural origins of human cognition. Cambridge, MA: Harvard University Press.
Vaesen K. (2012). The cognitive bases of human tool use. Behav Brain Sci, 35(4), 203-218.
Chapter 19 – Bird(songs) and Language
2019: Lynne Lee, Amanda Lim Li Ann, Chen Yi, Trudy Loo Soo May
1. Introduction
Language is the tool of communication that sets humans apart from animals. But what if animals could learn languages too?
Natural language is a language that develops naturally between humans through interaction (Lyons, 2006). Whether animals have the capacity for language or something comparable to natural language is widely speculated, with people citing evidence from animal communication in primates (Savage-Rumbaugh et al., 1980), birds (Pepperberg, 2002) and dolphins (Herman, 1986). Language experiments conducted on primates have also been carried out over the years with varying degrees of success – Koko the Gorilla, Washoe and Kanzi, just to name a few. These animals have shown that they are able to learn and use aspects of human languages to a certain extent. More recent language experiments have been conducted on birds to find out whether they have language and whether some of them might even be able to learn and communicate to the extent that humans do. This wiki chapter will look at several studies of communication among songbirds and grey parrots, and discuss whether it is appropriate to claim if their communication systems are developed to a degree similar to that of human languages.
Testing Wiki
Test
Test test test
4. Future Applications
Introduced in 2015, Skype’s auto-translate function serves to improve communication between people of different native languages by translating video calls in real-time.17 Imagine speaking with an online friend from halfway across the world, and as you see him on your computer screen, Skype rolls out sentence-by-sentence translations for whatever he is saying, like real-time subtitles.
It is a technology that breaks down language barriers between people. This has huge implications not just in a person’s social life, but also in making other areas of life such as work and travel much easier.
Granted, Skype’s auto-translations are not quite there yet and often fails to give accurate translations of utterances. But what is illuminating is how, just a decade ago, no one would have predicted that such technology would be made possible. Its mere inception is a reminder of how much room there is for development, and even though there are faults in the app’s language processing ability, we can be sure that with more research and improvisation, such faults might be ironed out in the future.
It has already been done with devices that are closer to us. Look at your mobile phone, or even your computer. Within it lies your own personal assistant. Take Apple’s Siri, for example. Its inception in 2011 was not quite successful. People were intrigued by its ability to chat, to answer questions, and to provide route directions. Yet, more often than not, Siri invoked a lot of frustration by misinterpreting commands.18
But that was in 2011. Now, because of rapidly evolving new methods of machine learning (such as deep learning and genetic algorithms, as we’ve discussed earlier), Siri is more intelligent. The difference is that Siri has been supercharged with artificial neural networks. And, according to Eddy Cue (below; inset), Apple’s Vice-President of internet software and services, it has “impacted all of Siri in hugely significant ways” such as its speech recognition, natural language understanding, execution of requests, and responses to the user. Because Siri now understands and uses language so much better than before, she has become an efficient digital personal assistant to whoever owns an Apple device, many of whom find that they cannot do without her in their day-to-day lives.
The repercussions associated with successful language processing abilities in machines are huge. When machines are able to grasp a language and use it well, they have the capacity to make our lives easier. The best thing is that the area of language learning in Artificial Intelligence is continuously evolving and expanding. In fact, one of the big predictions for AI in the year of 2017, according to MIT Review’s Will Knight, is that we can “expect further advances” in the area of language learning.19 It will be more than just voice recognition and obeying commands.
It may prove to be a formidable challenge, but how exciting it would be to finally have machines understand us in ways we never once thought possible.
3. Ways that computers can teach themselves to understand the human language
3.1 Machine programming
Earlier generations of artificial intelligence used the rules-based approach to program computers to learn and reproduce the human language. However, this has human limits as computers can only respond based on the algorithms they have been programmed with, or as they say, “Garbage in, garbage out”. Some language software use this rules-based approach to program the computer to compose sentences and semantically-correct phrases.
One example is LuaJIT, which is a scripting language designed and created by Tecgraf, a team at the Pontifical Catholic University of Rio de Janeiro (PUC-Rio). LuaJIT is able to support procedural, object-oriented, functional and data-driven programming as well as data description.9
The script for the video above was written using LuaJIT, using data in the form of previous scripts written by the host himself. The program processes all the data that has been inputted before creating its own script. However, while the software is able to construct grammatical sentences, it is unable to construct meaningful sentences that combine logical propositions using the rules-based approach. The end result is a sentence that is grammatically correct and logical in its parts, but ungrammatical nor illogical as a whole, complete sentence.
The way to progress beyond such limits is to develop the ability for machines to learn, in the same way that humans are able to learn, deduce patterns and infer possibilities.
3.2 Machine learning
Technological advances have made machine learning a reality. Machine learning refers to computer software that can learn autonomously beyond the algorithm that it has been programmed with.10 Through the use of pattern recognition, computers can learn from and make predictions based on available data. For example, the quality of language translations improved dramatically in 2007 when Google transited from a rules-based approach to a statistics-based one to train its translation engines.11
The dark side of machine learning is that programmers cannot control what the machine learns. In 2016, Microsoft experimented with Tay, an artificial intelligence chatbot that was designed to interact with millennials via Twitter and messaging apps Kik and GroupMe, so as to learn the way millennials spoke. Unfortunately, Tay ended up learning racist and Nazi phrases from the community it interacted with.12
3.3 Deep learning / Digital neural networks
The next bound is deep learning with digital neural networks. Modelled after brain neural networks, digital neural networks broadly comprise (a) neurons arranged in multiple layers, (b) connections between the processing elements (artificial neurons), and (c) the adaptive weights of each of these connections. Each weight indicates the relative importance of a particular connection. If the total of all the weighted inputs received by a particular neuron surpasses a certain threshold value, the neuron will send a signal to each neuron to which it is connected in the next layer. The network then learns through exposure to various situations, by adjusting the weight of the connections between the communicating neurons.13
Scientists from the University of Sassari and University of Plymouth developed a cognitive model composed of two million interconnected artificial neurons. The system is known as ANNABELL (Artificial Neural Network with Adaptive Behavior Exploited for Language Learning). From a “blank slate” starting point without any pre-coded language knowledge, ANNABELL has already demonstrated an ability to learn 500 new words – the language capacity of a four year-old.14
3.4 Genetic algorithms
Inspired by natural selection, genetic algorithms mimic the evolution of potential candidate solutions towards the optimal solution. Each candidate solution has a set of properties (its chromosomes or genotype, or bits) that are expressed in terms of a genetic representation which can be mutated and altered, and a fitness function. As the candidate solutions undergo iterative processes or generations of evolution, their genotype may be mutated or recombined, and the resulting fitness is evaluated.15
Genetic algorithms are used in natural language processing, including syntactic and semantic analysis, grammar induction, summaries and text generation, document clustering and machine translation.16 It has been found to be useful in the generation of dialogue phrases.
The development of artificial intelligence has been rapidly progressing as a result of these technological advances in machine learning, particularly in the domain of language processing. Computers are now exhibiting a heightened ability to convey and understand utterances in the natural languages. What could this mean for the big, bright future of AI? For now, let’s take a look at some applications that have already been invented and which play important roles in bettering our lives. Indeed, when machines understand language, humans are the ones who benefit most!
2. What a computer needs for it to learn and understand the meaning in language
2.1 It needs to understand the differences between utterances, sentences and propositions.
The spoken language can be broken down into three significant layers – utterance, sentence and proposition. The most concrete of the three is utterance which refers to the act of speaking. It involves a specific person, time and place but does not encode any special form of content. Utterances are identified as any length of speaking by a single person with distinct silence before and after. It need not be grammatically correct and can be meaningful or meaningless.
A sentence refers to the ‘abstract grammatical elements obtained from utterances’ .5 Unlike utterances, sentences cannot be defined by a specific time or place. They are grammatically complete and correct strings of words that express a complete idea or thought. An example of this would be if someone asked “Would you like a cup of tea?”. The reply could be “Yes, I would like a cup of tea.” or “No, I would not like a cup of tea.”. These two utterances can be considered sentences as they are both grammatically complete and correct and express a complete thought. However, if the reply was either “No, thank you.” or “That would be nice.”, they would be considered utterances but not sentences as the first is not grammatically complete and the second does not express a complete thought. The person who had asked the question, however, would still be able to perfectly understand the reply.
These non-sentence utterances play a large role in our day-to-day communication, and while they cannot be considered sentences, they still contain the abstract idea of a sentence. This brings us to our third layer of language, propositions. Propositions are concerned with the meanings behind the non-sentence utterances as well as whole sentences. It can be defined as the meaning behind an utterance and are claims or ideas about the world that may or may not be true.
As suggested by the Chinese Room Argument, computers are able to recognise utterances and sentences through a series of commands and are even able to respond accordingly. However, computers seem to face difficulty understanding and constructing propositions.
2.2 It needs to understand that the spoken language is able to encode meaning in different ways, such as patterns and unspoken meanings.
Speech is also able to encode meaning in ways that the written language cannot such as through tone, context, shared knowledge etc. It allows things to be left unsaid and indirectly implied. An example to demonstrate this would be if someone asks, “Have you finished writing your essay?” Your response could be “I started writing it but…” and end it there. While in the written language, a ‘but’ would indicate that there is more to come in the sentence, in the spoken language, the person would understand that the sentence is finished and fully comprehend the reply in association with the facial expressions conveyed with that utterance. True language comprehension requires computers to identify hidden and context-dependent relationships between words and clauses in texts.
2.3 It needs to have the ability to understand concepts, mental representations and abstract relations.
The semiotic triangle shows the relationship between concepts, symbols and real world objects. Together, they form the building blocks of language. Words contain representations of the world, as well as abstract, relational concepts embodied by the words. Concepts are the abstract ideas that these words represent. As such, they possess perceptual qualities as well as sensations through association. The word “dog” can possess the following representations: mammal, furry, barks, has a tail, man’s best friend, canine. The language processor must be able to know what each word represents, and be able to put it together to know what it is referring to even in the absence of immediate stimuli.
Insofar as a computer programmer can programme for the meaning of each word to be the multiple representations for each concept, similar to a dictionary, as well as the various grammatical and syntactic rules of language, then we can say that the computer would be able to learn the basic building blocks of the human language. This, however, limits the ability of the computer to the data and codes it has been programmed with.
2.4 It needs to have the ability to understand that others have different perspectives from itself.
As we have seen, language is very much influenced by thought. We can express words, sentences, utterances, but only if we are able to conceptualize them first. Do machines possess this mental aspect of language – the faculty of imagination and rational thought? It seems like the language that they know and use to communicate with one another, or with humans, is based upon only an algorithm.
This leads straight into the Theory of Mind (ToM), which is the ability to attribute mental states to oneself and others, and to understand that others have different perspectives from one’s own.6 The ToM has been found in non-human primates and humans, and is closely connected to the way we empathize with others. It explains our emotional intelligence as well as our intuitive desires to understand other humans and why they think the way that they do.
Our minds have sometimes been likened to machines (based on its abilities to process information, solve mathematical problems, and store important data), yet can we switch the nouns around to say that the machine functions like a mind? If we are on the side that is compelled to say that computers understand language, then we must say that they have a mind, since understanding language is a trilateral feat in which the mind plays an important role.
However, even though a computer may seem to understand our basic utterances that have their meanings entrenched in the structures of the world, it seems to meet measurable difficulty when it comes to understanding nuances. Aspects of speech like sarcasm, dry humour, and puns may not be understood by the computer unless they have been programmed to, and even this programming might require a technology far reaching beyond our times. But this reveals an important fact – sans programming, sans deliberate action in creation, the computer just cannot adapt to these aspects of human language.
2.5 It needs to have the ability to understand when different languages are being used.
Computer programmes can already identify what national language is being used through profiling algorithms. These pick out the words used in the text, and match them with the most commonly used words of a particular language, to identify what particular language is being used. It becomes trickier, however, when code-switching or hybrid languages such as Singlish or Spanglish are used. These mixed languages combine vocabulary, grammatical and syntactic rules from at least two different languages. In the case of Singlish, a relatively simple sentence could combine the lexicon and grammar from 6 different Singaporean languages and dialects. Decoding this would require a highly sophisticated computer, if at all possible.
2.6 It needs to adapt to the constantly changing and evolving spoken language.
Some linguists consider that the nuances contained in language make it impossible for computers to ever learn how to interpret. In addition, these nuances are not static but constantly evolving – application of these nuances rely not only on syntactic, phonetic and semantic rules, but also on social convention and current events.7 The word “bitch” could mean different things when spoken by different racial groups, in different social situations, and with different tones.
2.7 It needs to process speech, which has added complexity beyond written language.
It is difficult for computers to achieve good speech recognition. Spoken language differs widely from written language and there is wide variation in spoken language between individuals, such as differences in dialects.8 Researchers are looking into identifying what language is being spoken based on phonetics of the sounds of human speech. However, it is not as easy to distinguish phonemes and individual words when spoken, particularly when different speakers have differing accents, tonality, timbre and pitch of voice, pauses and pronunciations. One way to overcome this is through machine learning by providing massive amounts of data for the computer to discern patterns.
In the following section, we look at some of these methods of machine learning that have been developed to enhance the language comprehension skills of a computer.