转载整理自:http://www.wangdali.net/mic/
麦克风可以将声音的变化通过特定的机制转换为电压或者电流的变化,再交给电路系统进行处理。声音的强度,通过声压表示,对应电压或电流的幅值;声音变化的快慢,则对应电信号的频率。
声压级和距离的关系:
其中:Lp1表示距离为r1处测量的声压级,Lp2表示距离为r2处测量的声压级。
分类
根据不同的声电转换机制,麦克风分为不同的类型,包括动态麦克风、电容式麦克风和压电式麦克风等。由于输出信号比较微弱,一般麦克风都会配合前置放大器(Preamplifier)一起使用,再与后端电路连接。
http://hyperphysics.phy-astr.gsu.edu/hbase/Audio/mic.html#c1
http://www.wangdali.net/mic/
参数
http://www.analog.com/media/cn/technical-documentation/application-notes/AN-1112_cn.PDF
http://www.analog.com/en/analog-dialogue/articles/understanding-microphone-sensitivity.html
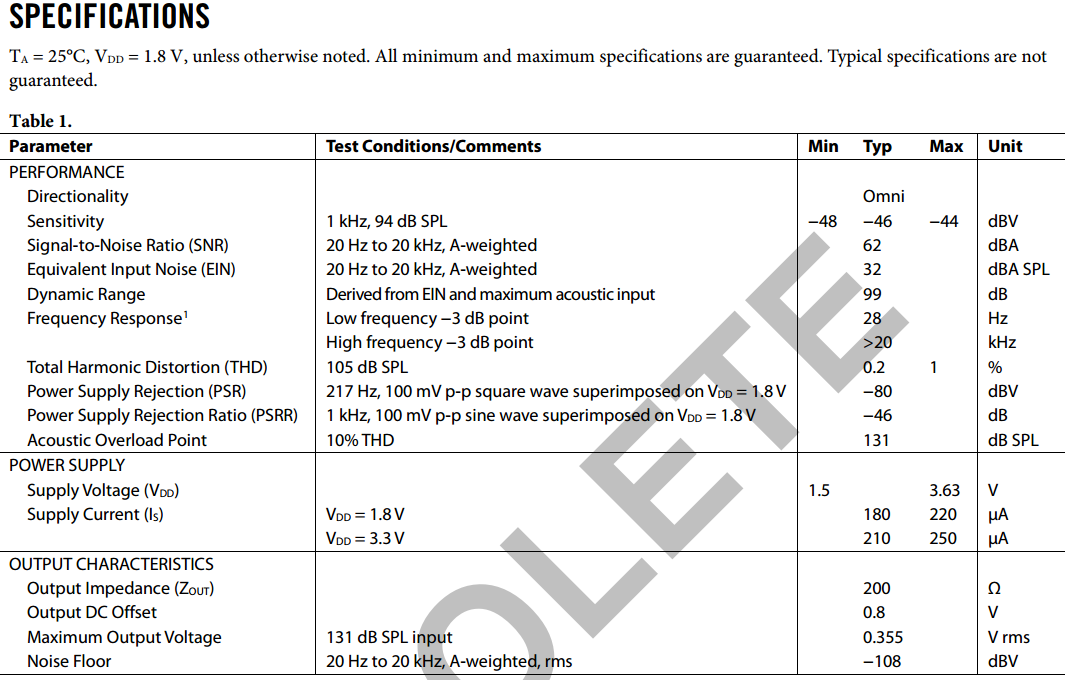
ADMP411 MEMS麦克风参数表
灵敏度(Sensitivity)
麦克风的灵敏度是指其输出端对于给定标准声学输入的电气响应。 用于麦克风灵敏度测量的标准参考输入信号为 94dB 声压级 (SPL) 或 1 帕( Pa, 衡量压力的单位) 的 1 kHz正弦波。 对于固定的声学输入, 灵敏度值高的麦克风比灵敏度值低的麦克风输出的电信号幅度高。 麦克风灵敏度(用dB 表示) 通常是负值, 因此, 灵敏度越高, 其绝对值越小。
务必注意麦克风灵敏度参数的单位。 如果两个麦克风的灵敏度不是采用同一单位来规定, 那么直接比较灵敏度值是不恰当的。 模拟麦克风的灵敏度通常用 dBV 来规定, 即相对于1.0 Vrms 的比值(dB)。 数字麦克风的灵敏度通常用dBFS 来规定, 即相对于满量程数字输出(FS))的 比值(dB)。 对于数字麦克风, 满量程(全“1”)是麦克风输出数字编码可以表征的最大值; 关于该参数更详尽的描述,参见”最大声学输入“部分。
灵敏度指输入压力与电气输出( 电压) 的比值。对于模拟麦克风, 灵敏度通常用 mV/Pa 来衡量, 其结果可通过下式转换为 dB 值 :

其中 OutputREF 为 1 V/Pa (1000 mV/Pa) 。
对于数字麦克风, 灵敏度表示为 94 dB SPL 输入所产生的输出占满量程输出的百分比。 数字麦克风的换算公式为 :

其中 OutputREF 为满量程数字输出水平(1.0)。
较高的灵敏度并不总是意味着麦克风的性能更佳。 麦克风的灵敏度越高, 则它在典型条件(如交谈等) 下的输出水平与最大输出水平之间的裕量通常也越小。 在近场(近距离谈话) 应用中, 高灵敏度的麦克风可能更容易引起失真,这种失真常常会降低麦克风的整体动态范围。
举例:
上表列出麦克风的灵敏度-46dBV,根据此参数换算输出电压与声压的关系:10^(-46/20) = 0.00501 V/Pa = 5.01 mV/Pa
输入声压比如120 dBSPL (20Pa) 的声音,麦克风的输出 = 5.01 mV/Pa * 20 Pa = 100.2 mV (RMS)
结合上文中公式,可以做个倒推计算,对于输出强度例如5.01 mV/Pa的麦克风,换算出其灵敏度:20 * log [(0.00501 V/Pa)/(1 V/Pa)] = -46 dBV // @94 dBSPL
附件为换算麦克风灵敏度的小工具:Mic Sensitivity and dB Convertor
信噪比(SNR)
信噪比(SNR) 表示参考信号与麦克风输出的噪声水平的比值。 这种测量包括麦克风元件和 MEMS 麦克风封装中集成的 ASIC 二者所贡献的噪声。 SNR 为噪声水平与标准 1kHz、 94 dB SPL 参考信号的 dB 差。
要计算 SNR, 须在安静、 消声环境下测量麦克风的噪声输出。该参数通常表示为 20 kHz 带宽内的 A 加权值 (dBA), 这意味着它包括一个与人耳对不同频率声音的灵敏度相对应的校正系数。当比较不同麦克风的 SNR 时, 必须确保它们采用相同的加权方式和带宽 ; 在较窄带宽下测得的 SNR 优于在整个 20 kHz 带宽下测得的 SNR。
动态范围(Dynamic Range)
麦克风的动态范围衡量麦克风能够做出 线性响应的最大SPL与最小SPL之差, 它不同于SNR( 相比之下,音频ADC 或 DAC 的动态范围与 SNR 通常是等同的)。
麦克风的 SNR 衡量噪底(EIN)与 94 dB SPL 的参考水平之差,但在该参考水平以上,麦克风仍然有相当大的有用信号响应范围。 麦克风能够对 94 dB SPL 至最高 120 dB SPL(即AOP) 的声学输入信号做出线性响应。 因此,MEMS 麦克风的动态范围等于其 SNR + 26 dB, 其中 26 dB = 120 dB(AOP) − 94 dB。 例如,ADMP404 的 SNR 为 62 dB, 而动态范围为 88 dB。
下图显示了声音输入(用 dB SPL 衡量)与麦克风电压输出(用dBV 衡量) 的关系。 动态范围和 SNR 显示于这两个刻度轴之间,以供参考。 图11利用 −38 dBV 灵敏度和 65 dB SNR的 ADMP504 来显示这些关系。
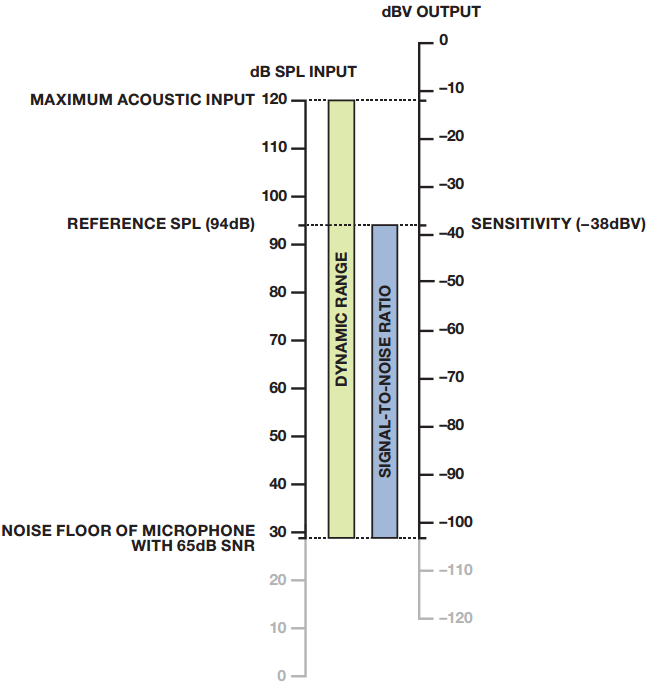
模拟麦克风的dBSPL输入与 dBV输出的关系
图12显示了数字麦克风的 dB SPL 输入与 dBFS 输出之间的类似关系。 注意, 在此图中,120 dB SPL 的声学过载点(AOP)映射 为 0 dBFS 输出信号。 只要声学过载点对应 0 dBFS 并且设置为 120 dB SPL, 则数字麦克风始终具有 −26dB 的灵敏度。 这是由灵敏度的定义(在 94 dB SPL 下测量)所决定的, 而不是可以通过改变麦克风 ASIC 的增益进行调整的设计参数。
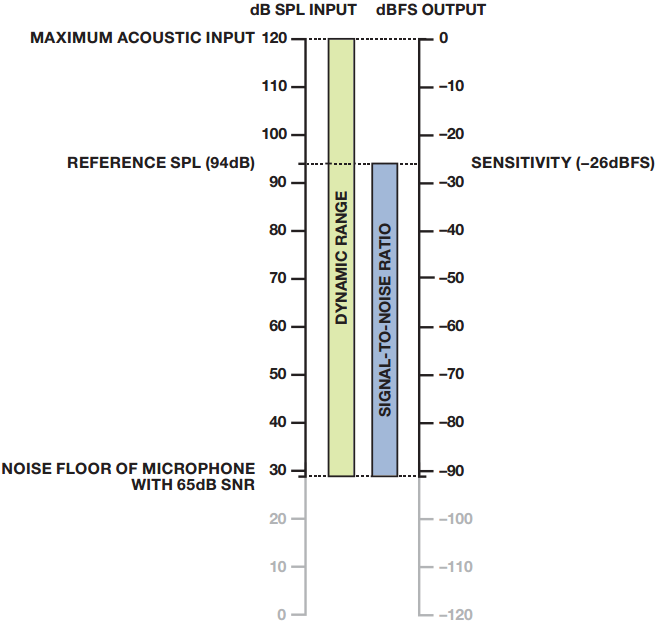
图12. 数字麦克风的dBSPL输入与 dBFS输出的关系
注:以上示例均假定麦克风的声学过载点(AOP)为120dB SPL
频率响应(Frequency Response)
麦克风的频率响应描述其在整个频谱上的输出水平。 频率上限和下限用麦克风响应比 1 kHz 的参考输出水平低 3 dB时的频率点来描述。 1kHz的参考水平通常归一化为 0 dB。
频率响应特性还包括通带内偏离平坦响应的限值。 这些值表示为 ±x dB, 说明 -3 dB 点之间输出信号与标称 0 dB 电平的最大偏差。
MEMS 麦克风数据手册用两幅图来显示此频率响应 : 一幅图显示频率响应模板, 另一个幅图显示典型实测频率响应。频率响应模板图显示整个频率范围内麦克风输出的上限和下限, 麦克风输出保证位于此模板范围内。 典型频率响应图显示麦克风在整个频段内的实际响应。 图13和图14的示例为选自 ADMP404 数据手册的两幅图。
图13. 频率响应模板
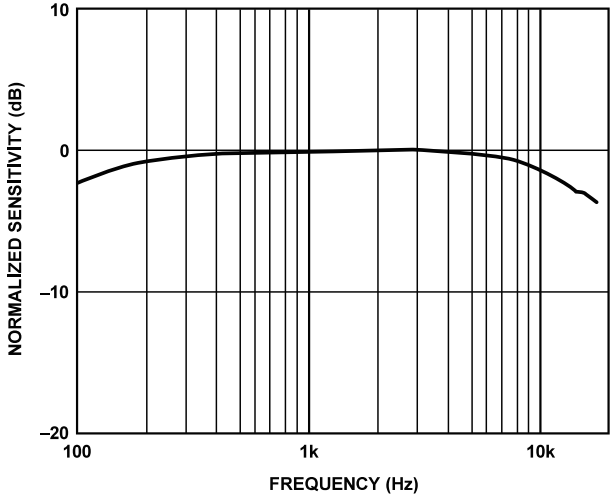
图14. 典型频率响应(实测)
频率响应较宽且平坦的麦克风有助于系统设计实现自然、清晰的声音。
总谐波失真(THD)
总谐波失真(THD) 衡量在给定纯单音输入信号下输出信号的失真水平, 用百分比表示。 此百分比为基频以上所有谐波频率的功率之和与基频信号音功率的比值。
ADI MEMS 麦克风的 THD 利用基波的前五次谐波计算。计算公式如下:
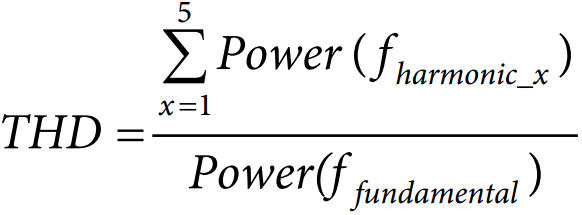
THD 值越高,说明麦克风输出中存在的谐波水平越高。
此测试的输入信号通常为 105 dB SPL, 比 94 dB SPL 参考高11 dB。 与其它参数相比, THD 在较高的输入 SPL 下测量,这是因为随着声学输入信号水平提高, THD 测量结果通常也会提高。 根据经验, 输入水平每提高 10 dB, THD 会提高 3 倍。 因此,如果在 105 dB SPL 时 THD 小于 3%, 则在95dB SPL 时 THD 将小于 1%。
注意不要将此参数与总谐波失真加噪声 (THD + N) 混淆,后者不仅衡量谐波水平, 而且包括输出中的所有其它噪声影响。
麦克风的线性度(Linearity)表征麦克风电信号输出幅度与输入声压的关系。图15和图16分别为ADMP411麦克风数据手册中THD和线性度的曲线图。
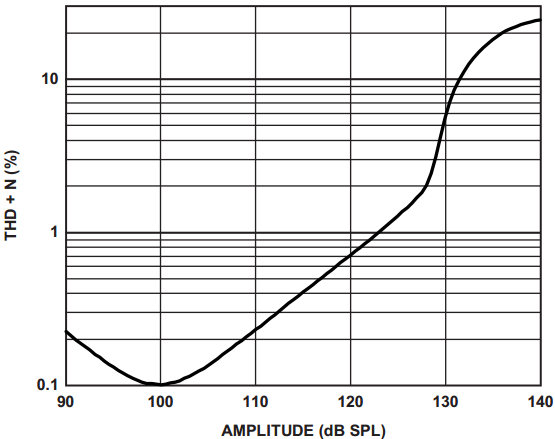
图15. THD + N vs 输入声压
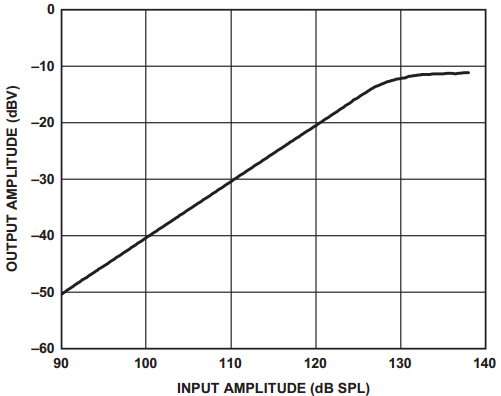
图16. 麦克风线性度
电源抑制(PSR)和电源抑制比(PSRR)
PSR: Power Supply Rejection
PSRR: Power Supply Rejection Ratio
电源抑制和电源抑制比是两个类似表征麦克风输出对于电源输入噪声抑制能力的参数。它们测量的是电源噪声影响到麦克风的输出的程度。测量方法有所不同。
电源抑制PSR通过将频率为100mV峰峰值,频率为217Hz的方波施加于麦克风的VDD引脚来测量。PSR的结果是在没有声音输入情况下,麦克风经过A-Weight滤波器,20kHz带宽的输出幅度大小。对于模拟麦克风,PSR的单位为dBV(负值);数字麦克风PSR的单位为dBFS(负值)。如果麦克风具有良好的电源抑制能力,则此PSR近似等于A-Weight滤波器的噪声水平。
电源抑制测量之所以使用217Hz频率, 是因为在 GSM电话应用中,217Hz开关频率通常是电源的一个主要噪声源。
电源抑制比PSRR测试使用的不是方波,而是频率为100Hz-10kHz,峰峰值100mV的正弦波施加到麦克风的VDD引脚。PSRR测量麦克风在频域内对电源噪声的抑制能力。测试PSRR的过程中,不需要使用到A-Weight滤波器。在麦克风的器件手册中,一般标定1kHz时器件的PSRR,图17表征ADMP510在100Hz-10kHz内PSRR参数曲线。曲线代表的含义为,在麦克风电源VDD引脚施以100mV峰峰值(-20dBV)的信号,在麦克风输出管脚测量到此频率信号输出值大小。输出信号的绝对值(dBV)要比图表中展现的dB单位的值低20(与输入-20dBV的比值,故减去20!)。
数字麦克风和模拟麦克风的PSRR参数曲线类似,数字麦克风电源抑制比单位为dBFS。不过数字麦克风的电源抑制比参数不是严格的”比值(Ratio)“关系,因为电源噪声输入单位为dBV(-20dBV),而麦克风输出噪声单位为dBFS,并非与电源噪声的比例关系。因此,数字麦克风的电源抑制比曲线中使用PSR,不同于本节第二段中的电源抑制参数(217Hz方波)。
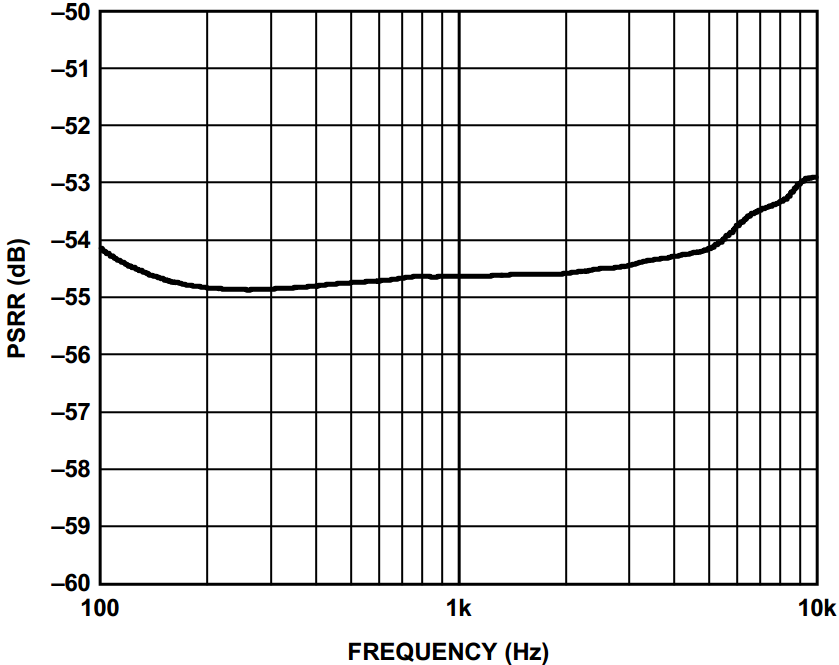
图17. 典型的PSRR与频率关系曲线(模拟麦克风)
表2列出了电源抑制PSR和电源抑制比PSRR参数的不同点。
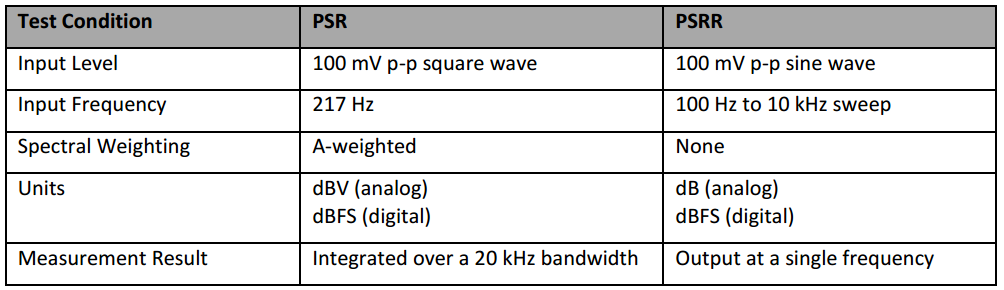
表2. PSR和PSRR的对比
声学过载点(AOP)
AOP: Acoustic Overload Point
声学过载点指的是麦克风输出THD等于10%时输入的声压大小(SPL),通常也称为麦克风的削波点。声压高于AOP的输入会造成输出信号严重失真。通过检测输出信号THD的波形决定AOP的大小,如图15所示。
AOP和THD的测试值并不能正确反映麦克风的输出随着失真增加变化的情况。无论是硬件或软件的削波,都会提供关于音质变化的额外信息。为表征麦克风输出随着SPL增加变化的情况,有些麦克风的数据手册会提供麦克风输出随着SPL增加在时域内变化的情况,图18表示输入不同声压的1kHz正弦声波,ADMP411输出电压变化的情况。
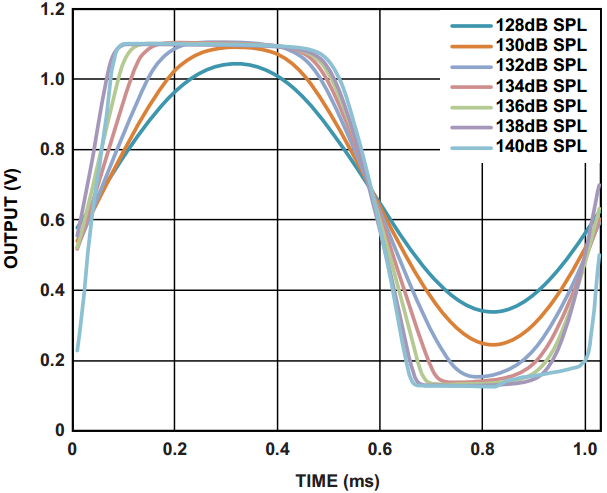
图18. 麦克风削波特性
Continue reading →